Using social networks data for GenAI training: unlawful by default or design?
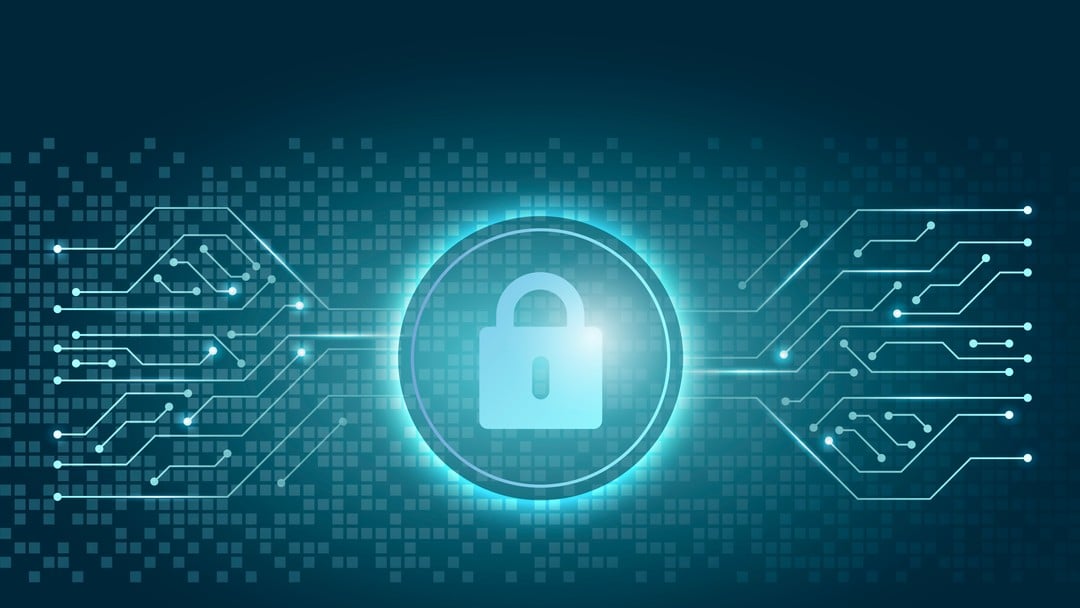
Dr. Ilia Kolochenko explores Meta’s pause on AI training with EU user content following GDPR complaints
On June 14, the Irish Data Protection Commission (DPC) welcomed Meta’s decisions to pause a previously announced training of Meta’s Large Language Models (LLMs) on user-produced content from Facebook and Instagram in EU/EEA states. Earlier in June, Meta informed its European users about the upcoming update of its privacy policy. The update would allow the social media mogul to utilize various user-produced content, such as users’ public posts, comments, and images, to train Meta’s AI technology. Meta adduced a legitimate interest as a lawful basis to process users’ data for AI training, while leaving a possibility to opt out by several convoluted steps.
Shortly after, noyb (acronym for “none of your business”) – the privacy advocating group led by Max Schrems – filed complaints in 11 EU member states against Meta for alleged GDPR violations. Meta would be better off taking these complaints seriously. Whether Meta’s decision was motivated by the complaints remains uncertain, but it is clear that the collection of user content for AI training is an arduous task amid the regulatory landscape.
In October 2023, the Confederation of European Data Protection Organisations (CEDPO) released guidelines “Generative AI: The Data Protection Implications”. The guidelines addressed key GDPR-related issues, a lawful basis for personal data processing and data subject rights (DSRs) whose data is used for LLM training. LLM models are composed of tokens and weights, being huge mathematical matrices. Models are capable of disclosing or generating personal data from training sets, in a misleading, inaccurate or even harmful manner. Various privacy-enhancing technologies (PETs) exist for data collection and cleansing, removing undesirable content from training data. However, even a comprehensive set of PETs cannot guarantee that personal data will not be inadvertently collected by LLMs. Some data cleansing techniques make AI training data worthless, as a model built on such quasi-synthetic data may be less intelligent or accurate, and more susceptible to bias, undermining the overall utility of the model.
LLM challenges
Right to be forgotten, right to rectify incorrect or outdated personal data, or the right to know how personal data is processed are almost impossible to be reliably exercised with LLM models. Fine-tuning a model can help reduce undesired processing and disclosure of personal data, but it does not guarantee that the model will not suddenly disclose a large portion of sensitive personal data due to overfitting or a creative command prompt attack. Additional safeguards can be placed between end users and a model, but these will not negate the potential for unlawful data processing or GDPR-related privacy violations. Ensuring accuracy of personal data collected from user interactions on social networks is difficult. Hate speech, defamatory, denigrating, copyright-infringing, or trademark-misusing comments may become part of an AI data training set despite filters and data-cleansing mechanisms.
But LLM privacy problems start here: even collection of accurate and truthful comments can cause unforeseeable and hazardous consequences. Imagine a comment from one user to another saying something innocent like “Happy Birthday my dear British friend, while I haven’t messaged you since Christmas dinner as you voted for conservatives, I truly admire your courageous fight with cancer and wish you a prompt recovery.” In one sentence, there is a set of sensitive personal data including political beliefs, religion, ethnicity and medical condition. Combining this with other public posts, comments or metadata of these two users can reveal a treasure trove of sensitive personal data.
Modern GenAI is good in object and text recognition on images or photos exacerbating the privacy dilemma. Millions of Internet users carelessly post documents, college transcripts, IDs, judgements or tax declarations on social networks. Even if Meta manages to obtain valid consent for processing of non-sensitive data, the consent may become insufficient and invalid for processing and later dissemination of sensitive information. Various open-source intelligence (OSINT) tools and frameworks have been available for a while for data mining of exposed personal data on social networks, but ongoing accumulation of all exposed personal data within an LLM model grants the model intrusive superpower and unlimited knowledge.
An opt-in consent may be futile to protect privacy and comply with law when users, who gave their consent for processing of their own personal data for AI training, unwittingly or purposely share or publish personal data of other users who never gave their consent for such processing. Many users discuss their friends, colleagues or celebrities on social networks, posting inappropriate content. With the surge of commercial spam bots and state-backed propaganda, the volume of deliberately false and misleading information on social networks becomes unthinkable. The victims of smear campaigns may even never have created an account on the social network in question. In sum, personal data on social networks is inseparable, making its processing for AI training purposes unlawful.
Compliance and privacy
Even if one day, social networks find a legally sound path to lawfully collect and process user-generated content for LLM training, theft of such model by foreign state actors or organized crime syndicates may have extremely perilous consequences. Threat actors may get the holistic and highly intrusive information about police officers, witnesses in criminal proceedings, journalists and other persons at risk. The information may include exposure of domicile and habits, enumeration of family members and friends, and other pieces of data that may lead to fatal consequences if misused. This is not to mention impersonation campaigns, when fake accounts artfully impersonate communication style of known persons for disinformation including attempts to influence financial markets, discredit politicians, or interfere with democratic elections. At the end of the day, a blindfolded race to maximize corporate profits with AI by social networks may erode everything from fundamental human rights to notional security.
Paradigmatically, GDPR is merely a tip of the EU legislation iceberg that has a direct and material impact on GenAI. The EU Digital Service Act (DSA), or the EU AI act scheduled to enter into force on August 20, erect an interviewed bundle of novel obstacles for Meta’s ambitions to train its LLM models on Facebook and Instagram users’ data. While some purely operational requirements of the above-mentioned EU legislation, such as record keeping, registration with authorities, maintenance of documentation, implementation of quality assurance systems, and performance of risk assessments – are comparatively straightforward to implement – others, for example, explainability of AI or corrective actions pose an almost insurmountable technical barrier. Another example is compliance with EU copyright law prescribed by the Article 53 of the EU AI Act. Every hour, social network users share millions of copyrighted texts, images and videos, sometimes unwittingly infringing copyright. Unless social networks manage to completely exclude content that infringes copyright from their AI training sets, the newly trained LLM models may pave the way for carpet-bombing copyright infringement litigation. While YouTube and other platforms have content recognition and watermarking systems to prevent upload of pirated content, the holes in their nets are still far too big, allowing a constant flow of copyright-infringing content to become publicly accessible for days, weeks or even years.
As a result, long-term economic viability of GenAI – without having a lawful access to high-quality and up-to-date data – remains highly questionable amid the progressively deflating GenAI hype and overall disillusionment. Possible liability for incorporating infringing, or otherwise unlawful, AI technologies into business processes spooks many potential corporate users of GenAI, who become increasingly savvier and better educated about AI, legality of underlying data collection and processing. Snowballing litigation on the other side of the Atlantic against both large and small GenAI vendors further corrode dreams of social networks to convert users’ data into digital gold. Notwithstanding that there is no overarching data privacy or AI legislation in the US, the FTC has been successfully policing the area under its famous Article 5 and reaching settlements with infringers requiring, among other things, deletion of unlawfully collected data, as well as destruction of algorithms and AI models trained on the data. Tellingly, the FTC is not a lone warrior taking care of unbridled use of GenAI, being followed by other federal and state agencies. While the recent Chevron decision by the US Supreme Court weakens rulemaking authority of federal agencies, Biden’s Executive Order14110 on AI will have long-lasting consequences on AI regulation in the US.
Conclusion
The rapidly intensifying AI regulation around the globe, in combination with existing and forthcoming privacy laws, make LLM training on social network users’ data largely uncertain and far too risky. Thus, the question before us is simple: whether such training is unlawful by default or unlawful by design, or, in other words, whether the patient is unsavable or there is a modicum of hope on the horizon. As always, time will judge.